Rapidly reducing the costs of carbon removal
There are various ways in which technologies have historically come down cost curves. We can harness lessons learned to bring CDR costs down more quickly.
This is a guest post by Grant Faber. Grant is the founder and president of Carbon-Based Consulting LLC, where he offers techno-economic assessment, market research, and other services to organizations working on carbon removal and decarbonization technologies. He has worked with organizations including XPRIZE, Heirloom, Twelve, and the Global CO2 Initiative and has published on various topics including learning curves for carbon capture and utilization.
Introduction
$100 per metric ton has become the “holy grail” of the carbon removal industry. Many view this as the cost target at which carbon removal becomes affordable at a massive scale, and it is the target chosen by the U.S. Department of Energy’s Carbon Negative Shot. The cost of permanent carbon dioxide removal (CDR) sets a ceiling on marginal abatement costs, bestowing significant economic importance on this figure. Even if $100/t is not feasible for many carbon removal approaches, every dollar counts: at 10 gigatons per year, each dollar per ton saved translates to aggregate savings of $10 billion per year. Clearly, cost is important, and it is incumbent on those working in the industry to help all carbon removal pathways come down their respective cost curves as quickly as possible.
How can we intentionally manage and accelerate this process? Many of us are already personally familiar with cost curves, which represent decreasing unit costs of a technology over time. Cell phones, desktop computers, flights, and many other modern conveniences are much cheaper today—when adjusting for inflation—than they were in the past. While not everything in our society has gotten cheaper and we are currently facing numerous inflation and supply chain woes, there is still something interesting at work here that is bringing down the costs of many goods and services that we might be able to harness and use to our advantage for CDR.
Many in the technology community will immediately think of Moore’s law when discussing cost improvements over time. Moore’s law is a famous observation by Gordon Moore—the founder of Intel—that the number of transistors on integrated circuits will double every two years, reducing costs in a similar manner. This was more of an observation than a physical “law,” and some speculate that it became a self-fulfilling prophecy by providing the industry with a target for improvement. Regardless, decreasing costs of computing changed our world dramatically, and the surprising stability of the trend is cited by many as the quintessential precedent for the exponential improvement of a technology over time.
Moore’s law ties performance, and thus cost, to time, but clearly it is not merely the passage of time that leads to better performance and costs. While time might be a proxy for or a predictor of improvements, there are different factors at play here occurring over time that we may be able to control. Many scholars have tried and are continuously trying to figure out what exactly drives performance and cost improvements. Greg Nemet, Jessika Trancik, J. Doyne Farmer, W. Brian Arthur, James Utterback, and countless others have made invaluable strides in describing the nature of technology and innovation. Lackner & Azarabadi and McQueen et al. investigate technological improvement as it specifically relates to direct air capture (DAC).
Through my review of these scholars’ work and my experience conducting techno-economic assessments (TEAs) of numerous technologies, I have created a typology that categorizes the primary drivers of cost reduction for emerging technologies. I describe this framework along with a case study of how it could apply to DAC in this post. Understanding these drivers is the first step toward figuring out ways to accelerate them, which the CDR community needs to do as much as possible to achieve our target of $100/t or beyond.
Typology of Cost Drivers
The chart above shows a breakdown of the primary drivers of technological cost reduction. The sections below discuss each driver in more detail.
Exogenous Cost Reduction Factors
Exogenous cost reduction factors arise from changes that are external to the technology under consideration. Said another way, they generally involve cheaper inputs, holding everything else about the technology constant. Changes to the costs of materials, energy, labor, transportation, waste disposal, permitting, equipment, construction, or capital all flow through to the final cost of whatever product they are used to make. Application of cost-reducing advances in other technologies would also be of an external origin. Government subsidies are also exogenous, as they are an artificial reduction in cost that is not directly related to the performance of the technology itself.
Even though something like cheaper materials might be exogenous to a particular technology, it is generally endogenous to another. A notable example of this could be how learning-by-doing—an endogenous cost reduction factor discussed below—has made electricity generated via solar photovoltaic (PV) systems much cheaper. This then serves as an exogenous, enabling factor for many other technologies that depend on cheap, clean electricity.
Endogenous Cost Reduction Factors
There are several endogenous cost reduction factors that derive from changing conditions for the technology itself rather than an external variable. These factors are likely the ones that most people think of when they consider technological improvement.
Economies of Scale (EOS)
Many in the business world might be familiar with economies of scale, which are reductions in unit cost derived from operating at larger production volumes. This is an endogenous factor as the reductions have a causal relationship with the scale of production, which is an inherent feature of the product of interest. Common EOS include volume discounts, distribution of fixed or semi-fixed costs (such as those for management or other administrative functions) over larger production volumes, sublinear cost scaling factors when using larger equipment, cheaper financing due to larger operations, and any other related efficiency gains. Other murkier examples might include agglomeration economies, workforce development, and more developed supplier networks, assuming they are related to the scale of the company or industry.
EOS do not continue indefinitely. At a certain point, there are diseconomies of scale where unit costs begin to increase again due to emergent complexity or market scarcity. As an extreme example to demonstrate this, one can imagine how it would be cheaper per car for a large firm to produce 100,000 cars instead of 1 car due to the level of fixed costs associated with this activity. However, if the firm were to try to produce 10 billion cars, they would very likely run into emergent logistical and supply issues that would begin to dramatically increase costs at a point, even though certain fixed costs would become negligible. Manufacturers should remain conscious of these, although they may not begin to apply until after market demand for a product is exhausted.
Learning-by-Researching (LBR)
Anyone working in deep/hard tech knows the importance of research for increasing the economic viability of emerging technologies. Funk & Magee specifically investigated the factors that lead to technological improvements and thus cost reductions when there is no commercial production and thus no potential to take advantage of economies of scale or other forms of learning. They found three primary mechanisms that contribute to improvements from LBR, which are “materials creation, process changes, and reductions in feature scale.” Respectively, these involve finding or inventing new materials that may cost less or have superior performance, using a faster or improved process, and reducing the scale at which a relevant process takes place.
Finding optimal combinations of materials and processes may also be a core part of LBR. Imagine a concrete scientist intentionally testing numerous combinations of materials using different mixing times, ratios, additives, etc. to identify the optimal mix. With time, the scientist might be able to develop a hypothesis about the superiority of different mixes or processes, thus deriving scientific truth from an empirical reality! This process of hypothesizing, testing, interpreting, and further hypothesizing is how the scientific method is translated into real technological gains.
Learning-by-Deployment
Learning-by-deployment is separated into learning-by-doing (LBD), learning-by-utilizing (LBU), and learning-by-interacting (LBI). This category represents advances in technologies that can be difficult to predict or ascertain without actual experience deploying the technology. Learning arising from deployment is generally difficult or impossible to predict or discover through research; if it were not, then you would not need to deploy to uncover these benefits.
LBD is the most well-known of the three. It is generally represented with “learning curves” or “experience curves” that most often demonstrate a decrease in unit costs with cumulative production. In place of Moore’s law, LBD is described with Wright’s law, whose eponym is Theodore Paul Wright, author of the classic 1936 article “Factors Affecting the Cost of Airplanes.” Wright’s law relates cost reductions to cumulative production rather than time, as this independent variable better captures the learning associated with manufacturing. Much ink has been spilled quantitatively describing LBD using learning rates, which are derived from Wright’s law and represent the percentage cost reduction with each doubling of cumulative production. A very popular example of learning rates is seen with solar PV; solar is widely calculated to have a roughly 20% decrease in the manufacturing cost per watt with each doubling of the amount of panels ever produced.
No one is quite sure exactly why LBD takes place. The concept of improving performance on routine tasks was noticed early in psychological studies, and most people can intuitively understand that they will improve their speed or performance to a point when doing the same tasks over and over again. In the world of technological production, scholars have uncovered various possible drivers for LBD, including process standardization, better equipment utilization, increased operating times, reduced waste/better material utilization, product redesign based on trial and error, automation, and workers learning on the job. While no one can fully explain why or how LBD occurs, we know it is extremely important for reducing costs. Structuring a business to maximize learning and allow for a high degree of trial and error can probably help accelerate this kind of cost reduction.
It has also been found that more complex technologies cannot take advantage of learning as much, likely due to the increasing number of combinations of different subcomponents that must be tried when undergoing trial and error. Less modular technologies or larger technologies also suffer here simply due to not deploying as many units; nuclear plants with negative learning rates are a famous example. Finally, technologies that are more central in patent networks have also been found to have higher rates of improvement, which may be related to more frequent use and thus more opportunities for learning.
The final two factors are learning-by-utilizing and learning-by-interacting. These can be somewhat vague but have been identified as relevant cost reduction drivers in literature. LBU is learning that arises from customers interacting with a product and giving feedback. There could be unnecessary features or different desired functions for a technology that, upon identification by customers, could be eliminated or added by the manufacturer in a way that reduces costs. It could be argued that customer or user interviews provide an early form of LBU.
LBI is learning that comes from interacting with supply chain partners. Many startups may experience high levels of LBI when they interact with established companies and receive advice on matters such as plant construction or commodity procurement. A common, early source of LBI might be through corporate partnership programs at startup accelerators.
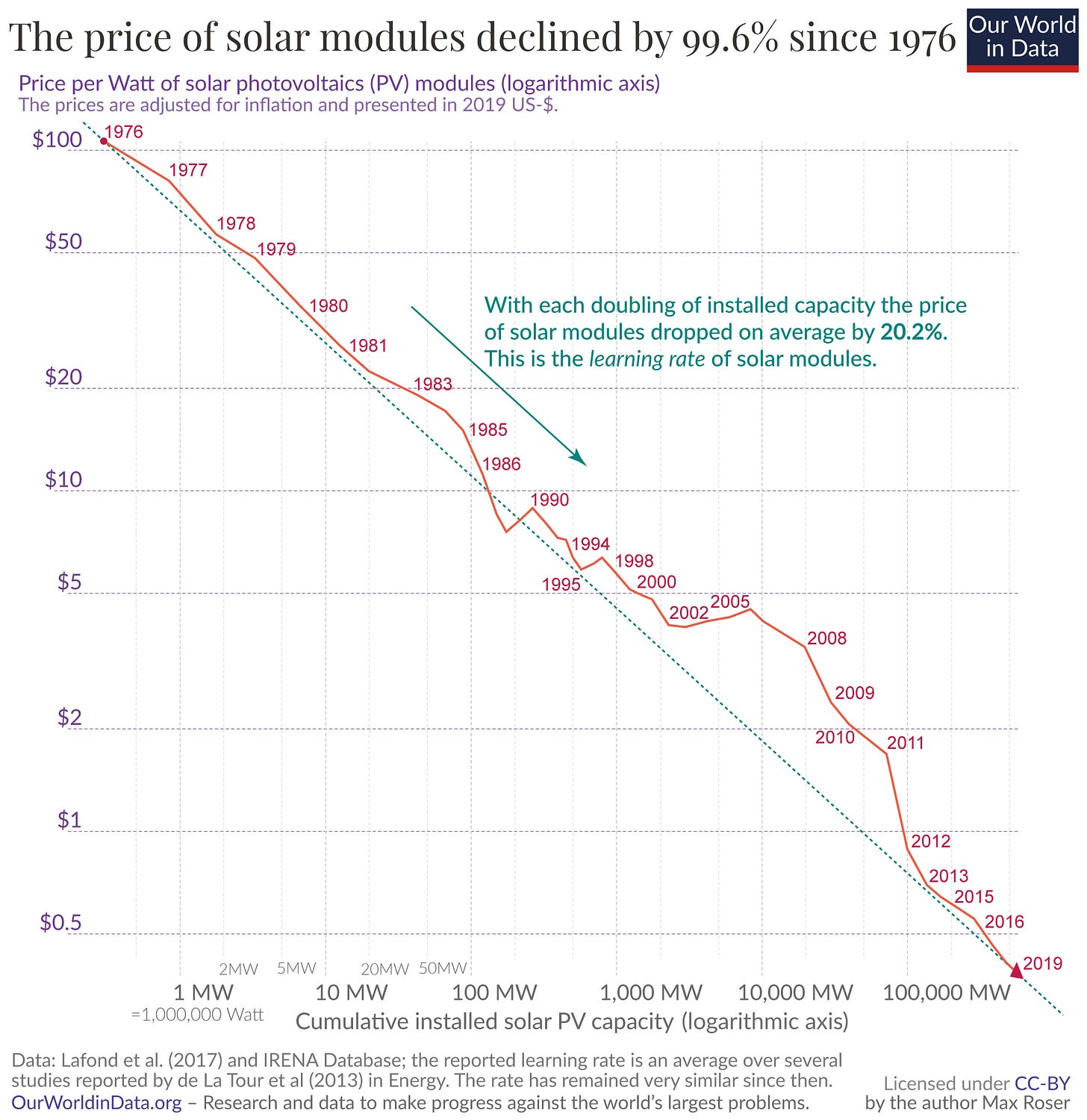
Further Considerations
There are several caveats that are important to be aware of when thinking about or using cost reduction factors. The first is that while these factors are often understood in terms of their direct impact on a technology’s cost, there can be indirect impacts on one factor from another.
For example, a government may decide to subsidize a technology through a tax credit, lowering the final cost for consumers via an exogenous factor. If investor confidence increases due to the increased likelihood of significant demand for the product, they may be willing to fund larger production facilities, allowing the technology to also take advantage of economies of scale. Larger plants would lead to more LBD over the same period of time, as cumulative production would be higher. Increased revenues for the firm could allow for more research funding as well, enabling LBR. One could argue in this case that government policy was the originator of all subsequent decreases, which is true, although analyzing cost reduction drivers in isolation helps provide more clarity about how they are reducing costs. However, it is incumbent on anyone who is commercializing a technology to take a holistic view of cost reduction and attempt to understand potential links and feedback between all factors.
Separately, it is important to understand that the relative importance of these effects generally changes throughout the life cycle of a technology. Scholars often indicate that LBR is more important in earlier stages and that EOS are more important in later stages, for example. It may be the case that certain opportunities are exhausted along a technology’s developmental trajectory, meaning that it becomes necessary to seek out other opportunities for improvement and cost reduction. Due to these differences, it may not be possible to neatly apply cost reduction factors for one technology or domain of technologies to another without considering the developmental stage, scope, and other relevant features of each technology.
Case Study: Direct Air Capture
This section provides a qualitative case study of how these cost reduction methods can apply to a generalized direct air capture (DAC) technology. The purpose is to quickly demonstrate how the methods can be immediately relevant to CDR and potentially inspire creative ideas about other creative cost reduction measures.
Exogenous factors could include cheaper clean energy, steel, concrete, input chemicals for sorbents, and capital. Advances in machine learning that enable better sorbent design would also be exogenous, as would government subsidies such as the 45Q tax credit.
Economies of scale might involve scaling plants to take advantage of sublinear cost scaling factors for CO2 compression, transportation, or sequestration equipment. If the plant is more modular in nature, economies of scale could apply to the manufacturing of the modules rather than the scaling of the capture or processing equipment. Bulk purchasing of raw material inputs and distribution of fixed or semi-fixed costs—such as those related to company management or even potentially non-recurring engineering costs—over larger capture volumes would also provide further economies of scale.
Learning-by-researching, which would occur to a greater extent during the initial stages or even before the formation of the company, might involve finding cheaper sorbents with faster cycle times, better desorption reactor designs, or more energy-efficient capture methods.
Learning-by-doing could arise from standardizing or automating processes at the plant to reduce labor needs, utilizing new and more efficient types of equipment for CO2 management, updating the configuration of the plant to increase throughput and reduce downtime, using better quality control methods over time to better identify and solve problems, or having increasingly skilled laborers better maintain the plant. As a side note, Climeworks experienced a notable instance of LBD with their novel sealing system, which was described during the launch of Orca.
Learning-by-utilizing is maybe somewhat vague in the case of a DAC plant as the customers might technically be those purchasing carbon removal offsets, although the “users” of the CO2 could also include those transporting or sequestering it. Such end users could better communicate their expectations over time for pressure, impurities, or some other feature of the captured CO2 in a way that saves cost for the DAC plant. Finally, learning-by-interacting could involve interacting with equipment or material suppliers to find new strategies for cost reduction. It could also involve finding better ways to interface with clean energy or energy storage developers to use clean energy more efficiently in the process.
Conclusion
Many working on carbon removal hope that costs will fall in similar ways to, if not faster than, other cleantech success stories to enable the massive scale of removals necessary to hit net zero and begin restoring our climate. This is not a given, but understanding the cost reduction factors can help illuminate the path forward.
How might the different drivers outlined here apply to your technology along its life cycle? Can you use this knowledge to your advantage? Is it possible to tie the success of your technology to some exogenous factor that is highly likely to come down in cost? Can you optimize your R&D process to more effectively focus on cost reduction? Can you design your process and company systems to minimize complexity and maximize learning? How can policymakers best support cost reduction?
Scholars and those commercializing new technologies consider related topics every day, and there is still much to learn about specific drivers of technological innovation. But even just attempting to answer these questions for your own work can help guide you in the journey of improving performance and reducing costs even more quickly, allowing the whole industry to further its goal of economically reaching gigaton scale.
To receive regular insights and analyses on carbon removal and the new carbon economy, please subscribe to this newsletter. If you enjoyed this post, please share it with friends. And if you’d like to get in touch with Na’im, you can find him on LinkedIn and Twitter.
Great read !
Wondering how could one apply this (cost reduction driver framework) to electrodialysis based ocean capture CDR ?